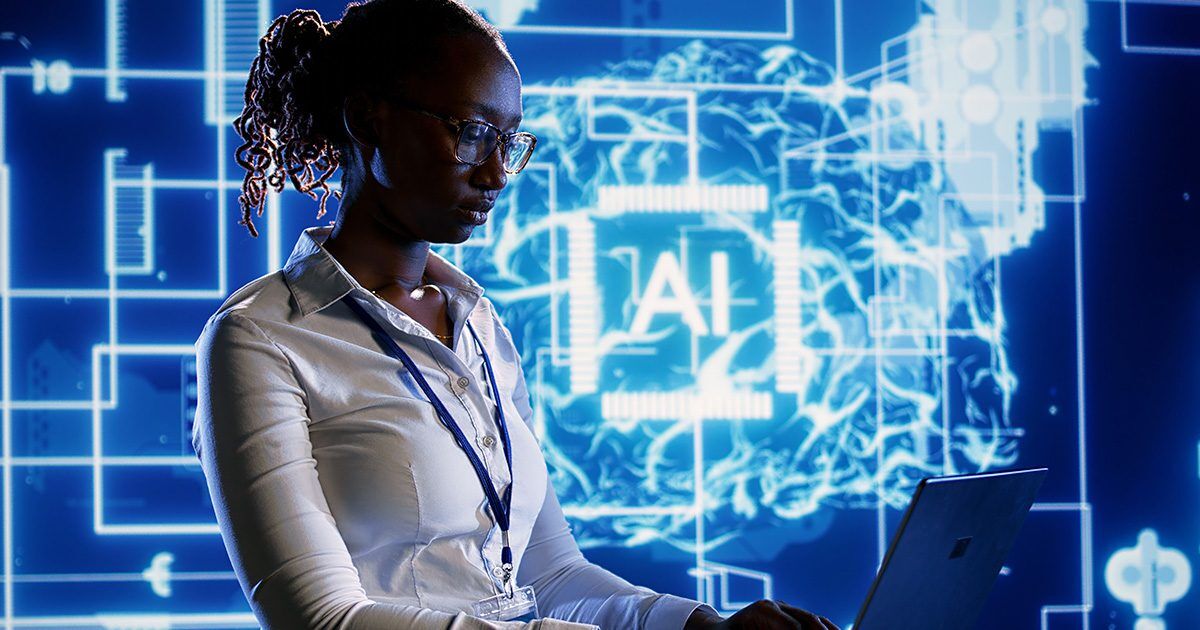
As the growth of artificial intelligence (AI) reaches into every facet of modern life, organizations everywhere are exploring new possibilities for leveraging the technology. AI applications range from relatively simple language translation operations to highly complex and time-sensitive medical diagnostics that could influence critical treatment decisions.
Each use case has specific parameters that must be met, and these parameters drive the infrastructure needed to support that workload. Your infrastructure choices can impact many things – performance, operational and management requirements, time to value, cost and more. The challenge is in understanding the options and tradeoffs in order to find the ideal solution.
Until recently, the GPU has been considered the go-to processor choice for AI and machine learning (AI/ML) performance for all workloads. But now there’s another option, a new generation of Intel® CPUs equipped with their own built-in accelerators – including Intel® Advanced Matrix Extensions (Intel® AMX), an instruction set that enables on-socket diverse AI and inferencing workload advantages – empowering an alternative for a wide range of use cases, as well as a host of additional benefits.
How do you know which processing option – CPU or GPU – is the best fit for a given workload? And how do you factor in cost, complexity, performance, time to market and other important issues? Presidio would like to share a few considerations when choosing CPU versus GPU for each AI use case.
Use case parameters and their impact on processor choice
Without dispute, GPUs are the sole option for large, complex AI workloads that exceed 13 billion dataset parameters, for datasets larger than 6GB, or for use cases that require fine tuning. The same holds true for complex functions that require sub-millisecond results for critical operations, such as parallel processing for AI training, or healthcare diagnostics.
In other words, not every AI task necessitates GPU-grade processing power. The starting point for considering CPU versus GPU is understanding your batch size, parameter requirements and response time requirements, as illustrated here.
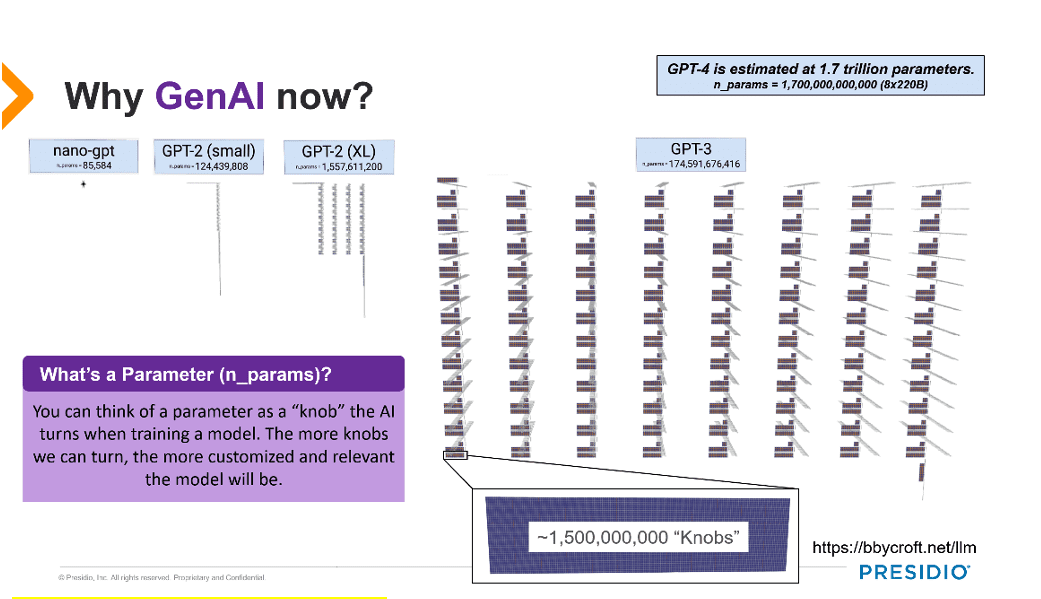
Comparing the benefits of a next-gen Intel® CPU versus GPU
Let’s say your parameters fall below the GPU threshold and you have flexibility in delivering time to results. It makes sense to explore the tradeoffs between a GPU and CPU to determine which one best satisfies your requirements and concerns.
The capabilities of the 5th Gen Intel Xeon Scalable processor make CPUs a viable choice for many AI use cases and workloads. One reason is the array of built-in accelerators in each core, including the Intel® AMX instruction set that enables an alternative for a wide range of AI workloads, improving efficiency without the need for a GPU. For many small- to medium-size AI workloads, this new CPU offers a sensible and cost-effective option – meeting performance requirements while offering numerous other benefits.
When evaluating a CPU vs. GPU for a given AI use case, consider these factors:
- Time to results – Will a few milliseconds of latency pose a mission-critical problem, or will it have no impact of any significance? If it’s the latter, then a CPU might be a more effective solution.
- Infrastructure pricing – GPU-based systems can be up to 3X as costly as comparable CPU systems, and that’s not even factoring in deployment costs, which are significant.
- Supply chain availability – GPUs can be difficult to procure, with long wait times for some high-end models, whereas latest-gen Intel Xeon Scalable processors are widely available.
- Scalability – Today’s GPU might not readily adapt to a changing environment, while these new CPUs are flexible and scalable enough to grow. And, as their model grows, customers who deploy with the Intel AMX instruction set can deploy Intel GPUs without the need to re-platform.
- Minimizing complexity – CPUs allow you to avoid code changes, reducing development time and promoting simplicity.
- Maximizing TCO and ROI – Because GPUs are extremely expensive and can be difficult to acquire and deploy, their operational costs can add up quickly. Moreover, they often consume more than 300 watts per GPU and have significant cooling requirements, directly impacting total cost of ownership and yielding diminished return on investment.
By including next-gen Intel CPUs in your AI strategy, select workloads can be processed within a reasonable time to value and at improved TCO and ROI.
CPUs help alleviate space constraints while promoting sustainability
Implementing sustainable practices is on the mind of virtually every management team, finding ways to do more with less in order to minimize environmental impacts. And that leads to a big drawback of GPUs: they consume significant energy resources for power and cooling – an environmental burden that impedes progress toward reaching sustainability goals. Moreover, GPUs require dedicated servers, exacerbating physical data center space limitations.
In contrast, 5th Gen Intel Xeon Scalable processors do not require specialized hardware or additional PCI-e lanes, they can function within existing data center footprints – and do so with significantly reduced resource consumption.
In short, AI-related workloads with more flexible parameters might be performed more efficiently and responsibly by the latest generation of processors with built-in acceleration.
CPU or GPU? Presidio can help you make the right choice
As the world embraces AI everywhere, technologies will evolve and expand to support its ubiquitous growth – including more processing options. The peril for many organizations lies in trying to implement an AI strategy alone – and failing in their initial attempt. They should understand that GPUs are no longer the sole choice for AI workloads, as next-gen CPUs present new alternatives for flexible performance as a viable alternative.
Because successful AI implementations are never “one size fits all,” Presidio works to modernize data centers and applications for each customer’s unique requirements. Whether your workloads are for AI inferencing, recommender systems, natural-language processing, generative AI or other applications, Presidio can help you measure your potential for deploying CPUs strategically to reduce TCO, right-size time to value, promote sustainability and improve ROI – all while maximizing your benefits from artificial intelligence.
Contact Presidio to discuss your AI use case and begin exploring the possibilities of utilizing a CPU versus GPU.